Cosma Shalizi May 6, 2019
Associate Professor in the Departments of Statistics and of Machine Learning at Carnegie Mellon University
Just How Doomed Is Causal Inference for Social Networks, Exactly?
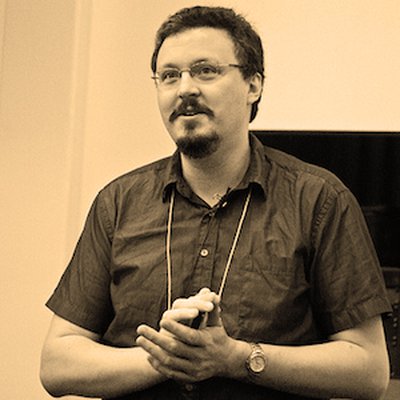
Yann LeCun November 8, 2018
VP and Chief AI Scientist at Facebook and Founding Director of the NYU Center for Data Science
Self-Supervised Learning
Deep learning has enabled significant progress in computer perception, natural language understanding and control. But almost all these successes largely rely on supervised learning, where the machine is required to predict human-provided annotations, or model-free reinforcement learning, where the machine learn actions to maximize rewards. Supervised learning requires a large number of labeled samples, making it practical only for certain tasks. Reinforcement learning requires a very large number of interactions with the environment (and many failures) to learn even simple tasks. In contrast, animals and humans seem to learn vast amounts of task-independent knowledge about how the world works through mere observation and occasional interactions. Learning new tasks or skills require very few samples or interactions with the world: we learn to drive and fly planes in about 30 hours of practice with no fatal failures. What learning paradigm do humans and animal use to learn so efficiently? I will propose the hypothesis that self-supervised learning of predictive world models is an essential missing ingredient of current approaches to AI. With such models, one can predict outcomes and plan courses of actions. Good predictive models may be the basis of intuition, reasoning and "common sense", allowing us to fill in missing information: predicting the future from the past and present, or inferring the state of the world from noisy percepts. One could argue that prediction is the essence of intelligence. After a brief presentation of the state of the art in deep learning, some promising principles and methods for self-supervised learning will be discussed.
Afsheen Afshar March 7, 2018
Chief AI Officer and Senior Managing Director, Cerberus
Real-world Challenges of using AI in the Enterprise
Recent advances in the field of AI has been exciting, and the resultant hype has been great. However, most enterprises have yet to substantively harness their data to positively affect their bottom lines. In this talk, we discuss some of the underlying technological and cultural reasons as well as approaches for success. From a technological perspective, there is a multitude of legacy systems with different formats and data models that must be merged. We discuss some approaches for managing this landscape using ad-hoc query methods. In addition, while technological and analytical challenges abound, having a high degree of cultural sensitivity, empathy for the end-user, and design-orientation are key to success. We discuss a few high profile examples of technologically advanced AI products that have failed to gain traction.
Stephane Mallat February 9, 2018
Professor, Ecole Normale Superieure, France
Learning Physics with Deep Neural Networks
Machine learning amounts to find low-dimensional models governing the properties of high dimensional functionals. This could almost be called physics. Algorithms have considerably improved in the last 10 years through the processing of massive amounts of data. In particular, deep neural network have spectacular applications, to image classification, medical, industrial and physical data analysis.
We show that the approximation capabilities of deep convolution networks come from their ability to compute invariant at different scales over possibly high-dimensional groups including diffeomorphisms. We shall study the mathematical properties of simplified deep convolutional networks computed with wavelet. We give applications to regression of molecular energies in quantum chemistry. We shall also introduce low-dimensional non-Gaussian intermittent models for statistical physics, with applications to Ising and high Reynold turbulences through cosmological data.
Peter Norvig October 25, 2017
Director of Research, Google Inc
Creating Software with Machine Learning: Challenges and Promise
Traditionally, software is built by programmers who consider the possible situations and write rules to deal with them. But recently, many applications have been created by machine learning: the programmer is replaced by a trainer, who shows the computer examples until it learns to complete the task. This shift in the way software is built is opening up exciting new possibilities and posing new challenges.